
Why Your Company Needs A Recommendation System
In today's digital era, it's essential for organizations to embrace advancements like Recommendation Systems. These systems have become fundamental in enhancing customer experiences and improving operational efficiency for B2B and B2C enterprises, mirroring the transformative impact seen in AI-driven technologies in recent years.
What Is A Recommendation System?
At its core, a Recommendation System is a subclass of information filtering systems that predict the preferences or ratings that a user would give to a product or service. Although Recommendation Systems are widely recognized for their role in B2C sectors like retail or entertainment, their integration in B2B scenarios is equally impactful, revolutionizing how businesses engage with other companies, optimize their operations, and make strategic, data-driven decisions.
Enhancing Customer Experiences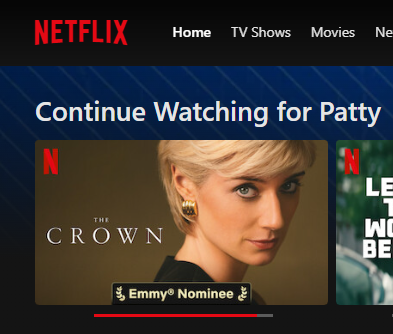
Recommendation Systems in a B2B setting have the unique capability to significantly personalize customer interactions. Trained on extensive datasets, they can analyze past interactions, purchase histories, and customer preferences to suggest highly relevant services or products, thereby enhancing the customer experience in a business-to-business context.
Driving Operational Efficiency And Data-Driven Decision Making
Recommendation Systems go beyond customer-facing applications. They are invaluable tools for internal business processes, aiding in decision-making and increasing operational efficiency. By analyzing patterns in large datasets, these systems can recommend process improvements, optimal resource allocation, and even guide strategic planning, offering insights that drive efficiency and effectiveness in various business operations.
Case Study: Salesforce Einstein AI In CRM
A prime example of a B2B application of recommendation systems is Salesforce's Einstein AI platform, used in CRM systems. Einstein AI uses machine learning and predictive analytics to score leads based on their likelihood to convert, predict sales trends, recommend personalized content, and provide customer insights. This has led to increased sales efficiency, enhanced customer engagement, data-driven decision-making, and operational efficiency for Salesforce users.
According to an IDC study, AI's integration in Salesforce is expected to generate more than $2 trillion in new business revenues between 2022 and 2028, with AI accelerating growth at a faster rate than previously predicted. This growth will also contribute to job creation, with a net gain of over 11 million jobs expected in the same period. These figures represent the significant economic influence of AI in enhancing Salesforce's cloud solutions and their broader economic impact. For more details, you can view the full article on the Salesforce website.
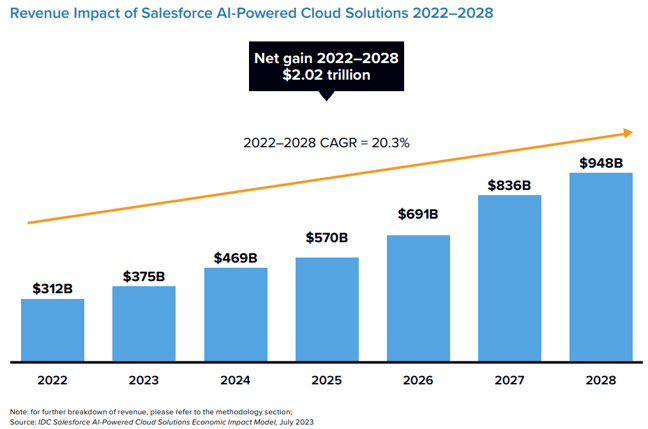
Types of Recommendation Systems
- Collaborative Filtering
- Content-Based Filtering
- Hybrid Recommendation
- Reinforcement Learning
- Context-Aware
- Demographic-Based
- Knowledge-Based
I'll explain each of these systems and give some real-world examples from services used by millions of people. You'll probably recognize some of these recommendation systems used at Netflix, Spotify, Amazon, YouTube, Uber Eats, LinkedIn, and TripAdvisor.
1. Collaborative Filtering At Netflix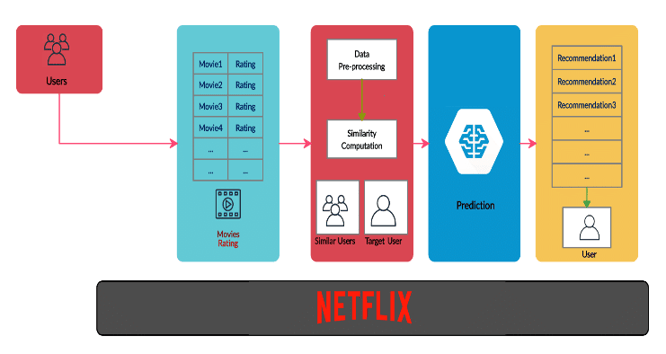
Collaborative Filtering uses the past behavior of a group of users to make recommendations to an individual. It assumes that if users agreed in the past, they will agree in the future.
Netflix analyzes the viewing habits of large groups of users and then suggests shows and movies that users might like based on what similar viewers have enjoyed. If you're a Netflix subscriber, you see these suggestions every time you open Netflix. You can learn more on "Deep Learning for Recommender Systems" in this Netflix case study.
2. Content-Based Filtering At Spotify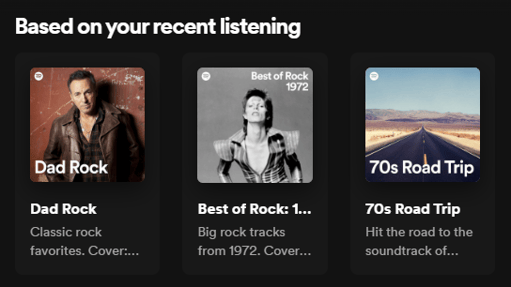
Content-Based Filtering: Uses item features to recommend additional items similar to what the user likes, based on their previous actions or explicit feedback.
Spotify uses content-based filtering for music recommendations. It analyzes the characteristics of songs users have listened to (like genre, artists, beat) and suggests new songs with similar features.
3. Hybrid Recommendation Systems At Amazon
Hybrid Recommendation Systems combine both collaborative and content-based filtering to improve recommendation quality and overcome the limitations of either approach when used independently.
Amazon combines collaborative filtering (based on user purchase and browsing history) with content-based filtering (considering the characteristics of products you've shown interest in) to provide personalized product recommendations.
4. Reinforcement Learning Recommendation System At YouTube
Reinforcement Learning Recommendation Systems use reinforcement learning to optimize recommendations based on user feedback, constantly improving based on actions like skips or plays in a music app.
YouTube uses reinforcement learning for its video recommendations where its system learns from user interactions (like video watches, likes, and skips) to constantly improve its recommendations.
5. Context-Aware Recommendation Systems At Uber Eats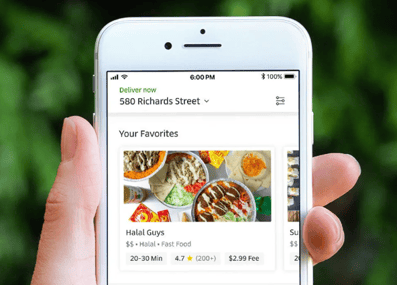
Context-Aware Recommendation Systems consider factors like location, time, weather, and device type to make situation-specific recommendations, such as suggesting food items on a delivery platform based on current conditions.
Uber Eats considers the user's location, time of day, and past ordering history to suggest relevant food choices.
6. Demographic-Based Recommendation Systems At LinkedIn
Demographic-Based Recommendation Systems make recommendations based on demographic data like age, gender, or location, assuming that people with similar demographics will have similar preferences.
LinkedIn offers job recommendations based on demographic information like job history, skills, and location in users' profiles.
7. Knowledge-Based Recommendation Systems At Tripadvisor
Knowledge-Based Recommendation Systems rely on domain expertise to recommend items, considering user requirements and preferences. For instance, in a hotel booking platform, they might suggest hotels based on a user's desired location, budget, and amenities, aligning closely with specific user needs and preferences.
Tripadvisor suggests hotels, restaurants, and attractions based on user preferences such as budget, location, and interests.
A Competitive Advantage In A Data-Driven World
Staying ahead means not just accumulating data, but also deriving actionable insights from it. A Recommendation System, like Salesforce's Einstein AI, provides a strategic advantage by allowing companies to leverage their data effectively, ensuring they remain competitive and relevant in a rapidly evolving digital landscape.
The Future Is Personalized
The future of B2B and B2C interactions lies in personalization. Just as Generative AI has made its way into public cloud offerings, making advanced AI technologies accessible, Recommendation Systems democratize the ability to offer tailored experiences and solutions in the B2B sector. They represent a shift from one-size-fits-all to a more nuanced, client-specific approach.
Recommendation Systems - A Key Strategy For Driving Growth
Adopting Recommendation Systems is a key strategic choice, reflecting a shift towards smarter, more efficient, and tailored business approaches. These systems are essential for enhancing customer experiences, driving growth, and improving operational efficiency.
At DesignMind, we understand the pivotal role of such systems in today's data-driven environment. Our expertise in AI and Data Science solutions, including AI Strategy and Roadmaps, Machine Learning and AI Model Development, and Large Language Models (LLM), position us to help your organization navigate and integrate these transformative technologies.
Connie Yang is Principal, AI and Data Science at DesignMind. She is an accomplished AI and Data Science leader with a strong background in data engineering. Learn about DesignMind's AI and Data Science solutions.